Machine Learning Solutions: Future of AI-Driven Innovation
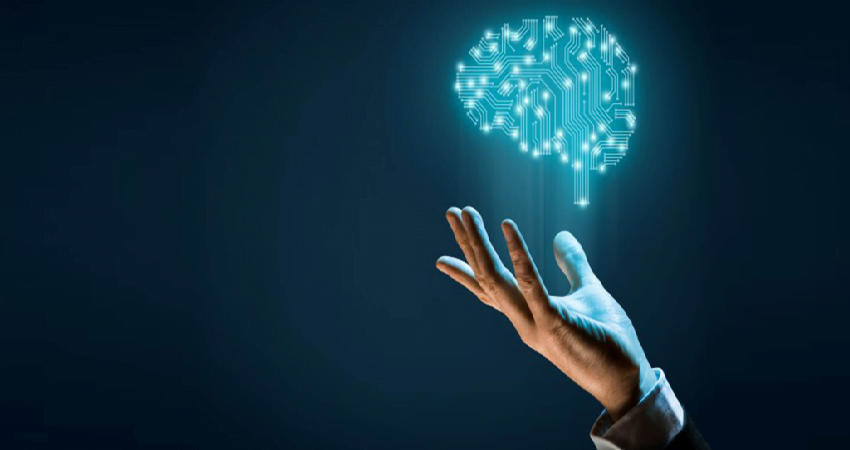
A machine learning solution encompasses a comprehensive set of intellectual assets, tools, and software designed for AI development across diverse devices. It empowers various ML techniques essential for AI, including supervised, unsupervised, semi-supervised, and reinforcement learning.
At its core, ML enables systems to learn from data and make decisions or generate outcomes based on inputs. However, the rapid pace of innovation can render machine learning solutions fragmented and outdated, suitable for specific devices or environments but not universally adaptable, leading to significant complexity.
A sustainable machine learning solution fosters AI development through a unified software framework, ensuring scalability, flexibility, and energy efficiency across a range of cloud and edge computing environments.
What Makes Machine Learning Solutions Significant?
Comprehensive machine learning solutions that commence at the device level are vital due to the evolving nature of the “machine” in ML. Machine learning solutions must be accessible across a spectrum, spanning from centralized data centers to the farthest reaches of a network, encompassing smartphones, fitness devices, heavy machinery, and predictive maintenance sensors. machine learning solutions establish a foundation for consistent and enduring development across a variety of ecosystems and applications, such as data processing on low-power devices within IoT or edge environments.
Top Machine Learning Solutions
1. Automated Machine Learning (AutoML)
Automated Machine Learning stands poised to transform the landscape of model construction in machine learning. In the past, crafting a machine learning model demanded the expertise of data scientists, domain knowledge, and substantial coding proficiency. Yet, AutoML empowers individuals without coding backgrounds to harness the potential of machine learning. It streamlines feature engineering, model selection, and hyperparameter tuning, democratizing machine learning and expanding its reach to a broader audience.
2. Explainable AI (XAI)
In an era of increasingly intricate machine learning model, comprehending their decision-making mechanisms is of utmost importance. Explainable Artificial Intelligence (XAI) strives to offer transparent and understandable rationales for the predictions generated by machine learning models. XAI fosters trust in the technology and equips stakeholders with insights into the reasoning behind a decision, elucidating both the “why” and “how” of the process.
3. Federated Learning
Amid growing apprehensions regarding data privacy and security, federated learning is emerging as a prominent solution. It enables the training of machine learning models across numerous decentralized devices or servers while safeguarding raw data. Instead, solely model updates are exchanged, guaranteeing data privacy. This methodology proves especially valuable in sectors like healthcare and finance, characterized by the presence of sensitive data.
4. Edge Computing and Machine Learning Synergy
The swift proliferation of Internet of Things (IoT) deployments necessitates streamlined and immediate processing of machine learning solutions at the network’s periphery. When edge computing is coupled with machine learning prowess, data analysis occurs directly on the devices, obviating the necessity for data to traverse to the cloud. This reduction in latency enhances privacy and augments real-time decision-making abilities. In the upcoming year, 2023, we anticipate notable strides in the convergence of edge computing and machine learning.
5. Reinforcement Learning (RL)
Reinforcement Learning (RL) stands as a subdivision of machine learning dedicated to acquiring optimal actions through iterative experimentation. Through the utilization of reward and penalty mechanisms, RL algorithms can acquire intricate strategies within dynamic and unpredictable settings. Given the recent progress in RL algorithms, we anticipate an expanded array of practical implementations across domains such as robotics and industrial automation.
Benefits of Robotic Process Automation (RPA)
RPA offers your enterprise the opportunity to streamline operations and minimize time-consuming human errors. RPA software functions by setting up automated bots to execute tasks typically handled by humans. These bots can be trained to navigate and input data across various applications and can also be programmed for intricate tasks, all without requiring human involvement. Automating a diverse range of functions, encompassing tasks like data transfer, customer profile updates, data input, inventory management, and even more complex operations with the benefits of robotic process automation.
Key Benefits of Robotic Process Automation (RPA):
- Time Savings: RPA automates repetitive tasks, freeing up time for more complex activities.
- Improved ROI: RPA enhances work productivity, leading to a positive impact on return on investment (ROI). It generates valuable data for cost management.
- Error Elimination: RPA ensures precise, error-free task execution, avoiding human errors and fatigue.
- Enhanced Security: RPA reduces human interaction with sensitive data, fortifying cybersecurity and preventing data breaches.
- Compliance Assurance: RPA ensures consistent adherence to industry and government regulations, reducing compliance risks and simplifying audits for various workflows and notifications.
- Scaling through RPA: Expanding automation across the organization for increased operational efficiency.
- Enhanced Employee Focus: Automation of repetitive tasks boosts employee satisfaction, enabling them to concentrate on strategic functions like business planning and PR.
Machine learning solutions and robotic process automation (RPA) are pivotal in shaping the future of AI development and business optimization. Machine learning solutions provide a unified framework for AI development, allowing scalability and adaptability across diverse devices and ecosystems. Automated Machine Learning (AutoML) democratizes ML by simplifying model construction, while Explainable AI (XAI) enhances transparency in decision-making.